Using AI for early,
accurate intervention.
How it works
Metadvice’s seamless integration with electronic medical records (EMRs) enables key capabilities that streamline workflow, increase health system capacity and support clinicians’ interactions with patients.
The Metadvice platform works in three steps:
By stratifying patient groups, Metadvice ensures the efficient allocation of resources. This strategic approach not only delivers timely and precise healthcare, it also increases capacity for early intervention – essential in chronic disease management.
Neural networks in action
The current situation
- ~50% of patients are not treated to guidelines
- Even when treated to guidelines ~50% don’t derive optimum clinical benefit
The Metadvice approach
Our neural networks are first trained to meet clinical guidelines. Once trained, they then assess the clinical responses of patients, first using synthetic data, and then using real-world data. From this assessment, the neural networks are able to determine in whom the guidelines work best, and in whom they are less effective. Using digital twin approaches, we are able to identify the most appropriate treatment options for individual patients.
Neural networks development
Clinical module
Continuous
learning
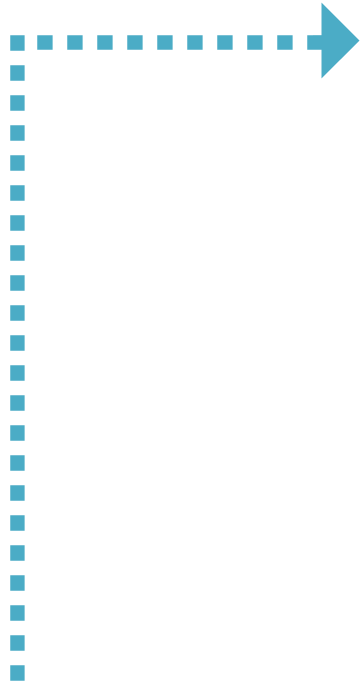
Training to guidelines
using large complex synthetic datasets and small real-world datasets
1a. Training
refine
1b. Testing
refine
1c. Validation
Medically knowledgeable neural networks able to provide a
response with ~98% accuracy to guidelines
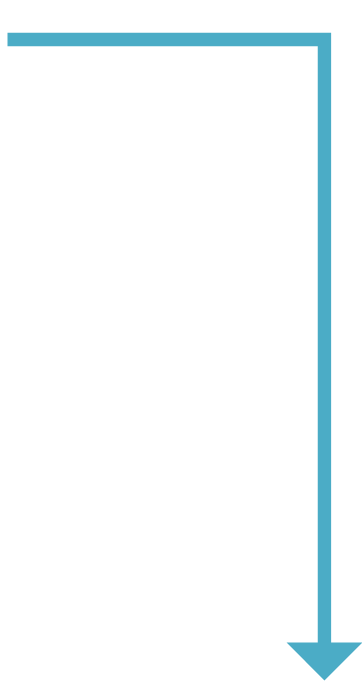
Provide actionable insights for the optimum treatment pathway
Determine the typical course of action for an individual according to guidelines
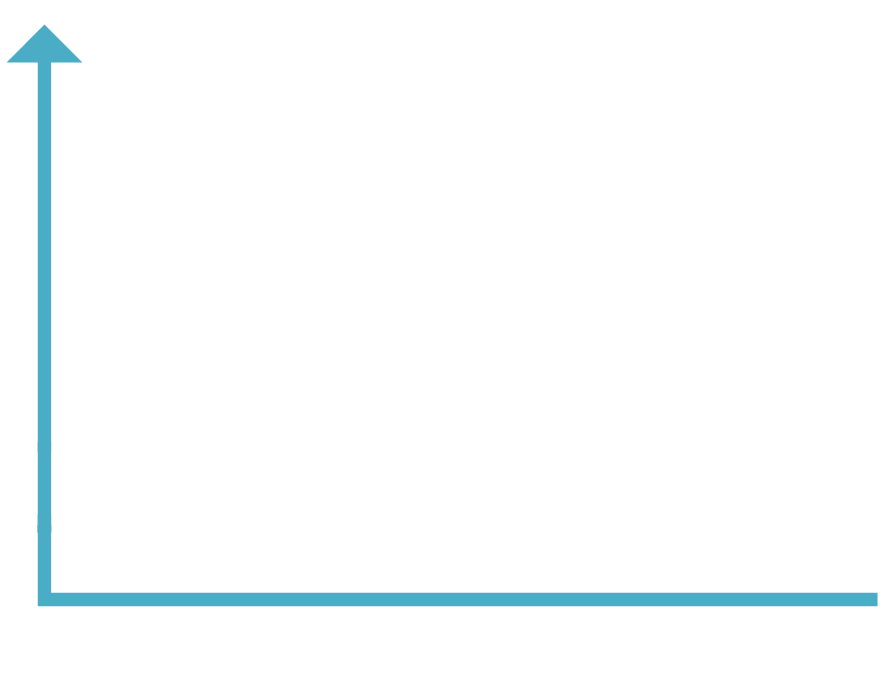
Transfer learning
Uses real-world evidence to assess
the most effective treatment pathways for different subgroups
2b. Digital twin cohorts
2a. Advanced learning
Identifies the appropriate course of action for subgroups
where guidelines do not achieve target outcomes
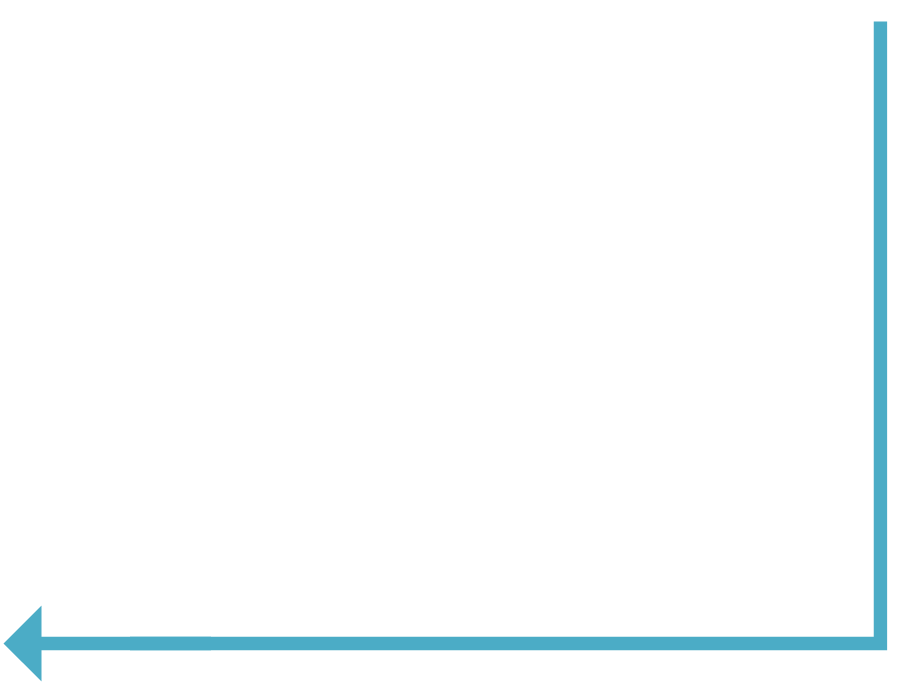
Continuous
learning
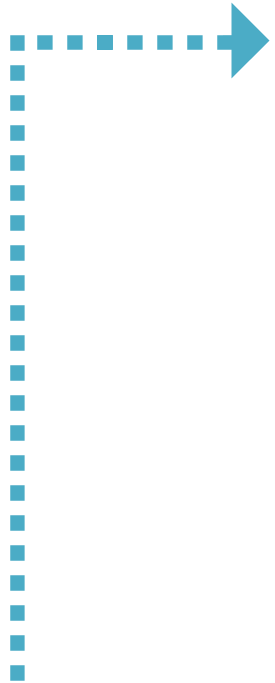
Training to guidelines
using large complex synthetic datasets and small real-world datasets
1a. Training
refine
1b. Testing
refine
1c. Validation
Medically knowledgeable neural networks able to provide a
response with ~98% accuracy to guidelines
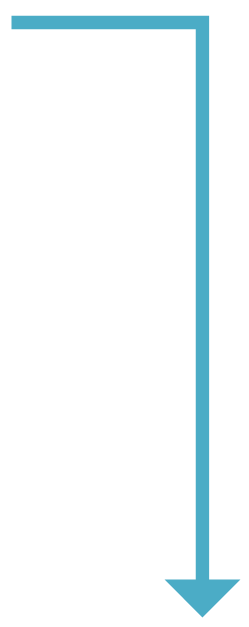
Provide actionable insights for the optimum treatment pathway
Determine the typical course of action for an individual according to guidelines
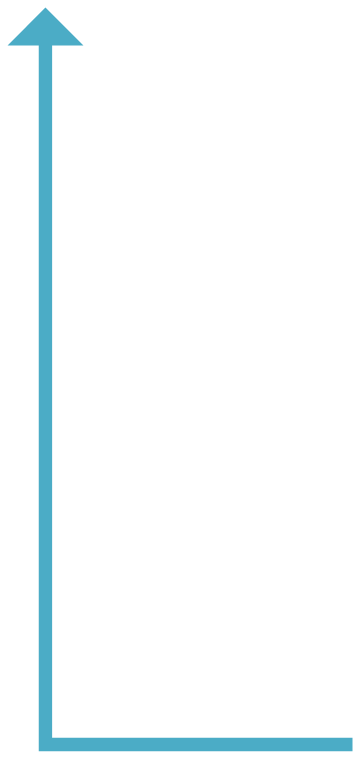
Transfer learning
Uses real-world evidence to assess
the most effective treatment pathways for different subgroups
2b. Digital twin cohorts
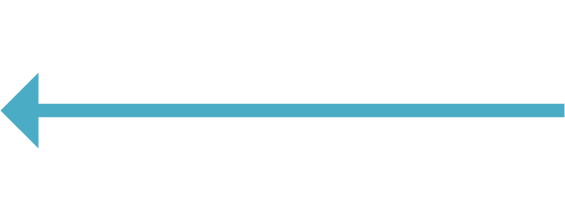
2a. Advanced learning
Identifies the appropriate course of action for subgroups
where guidelines do not achieve target outcomes
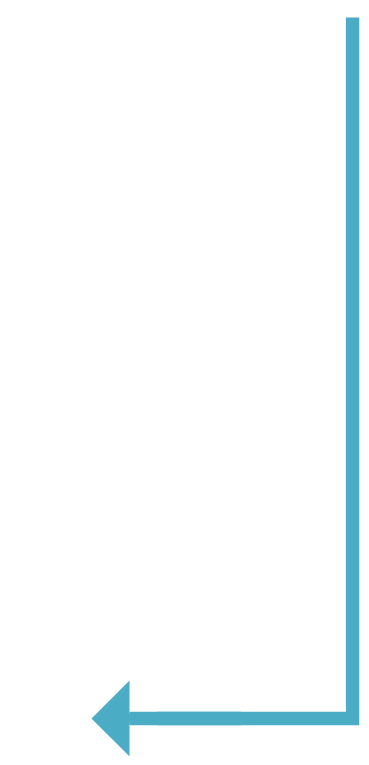
View on a larger screen for full explanation
Training to guidelines
1a. Training
refine
1b. Testing
refine
1c. Validation
Provide actionable insights for the optimum treatment pathway
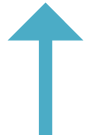
Transfer learning
2b. Digital twin cohorts
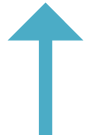
2a. Advanced learning
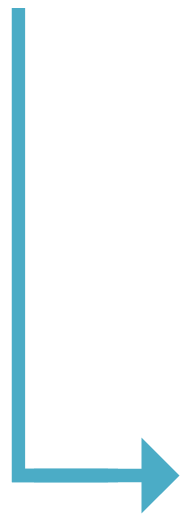
Determine the typical course of action for an individual according to guidelines
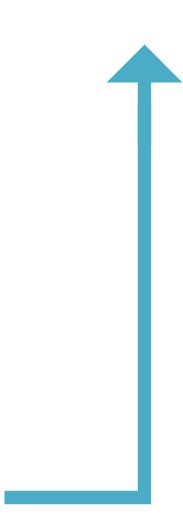
Comorbidity management
Cardiometabolic disease
Diabetes
Cardiovascular disease
Chronic kidney disease
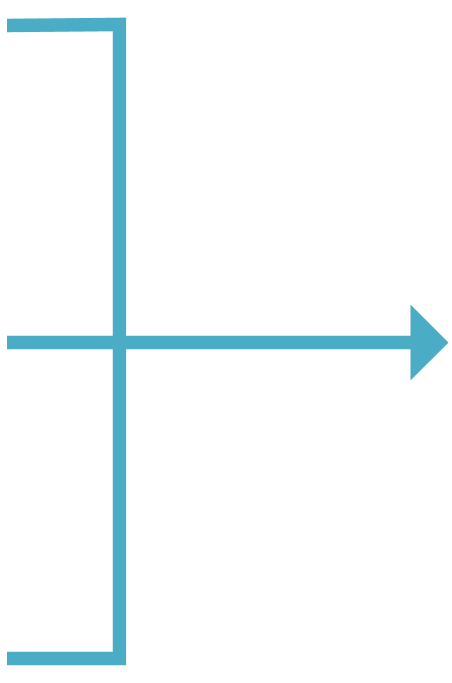
Uses real world evidence to assess most effective treatment pathways based on comorbidities
Synthesises combined outputs and prioritises treatment pathways, supported by statistical analysis
learning
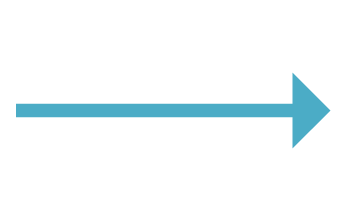
cohorts
Stratifies patients by overall clinical outcomes
Provides a nuanced approach for patients with comorbidities
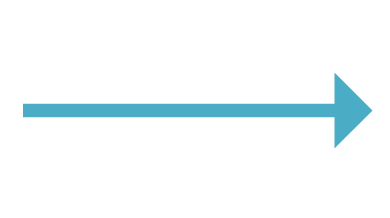
Neural networks able to provide optimal treatment recommendations based on individual profile
Diabetes
Cardiovascular disease
Chronic kidney disease
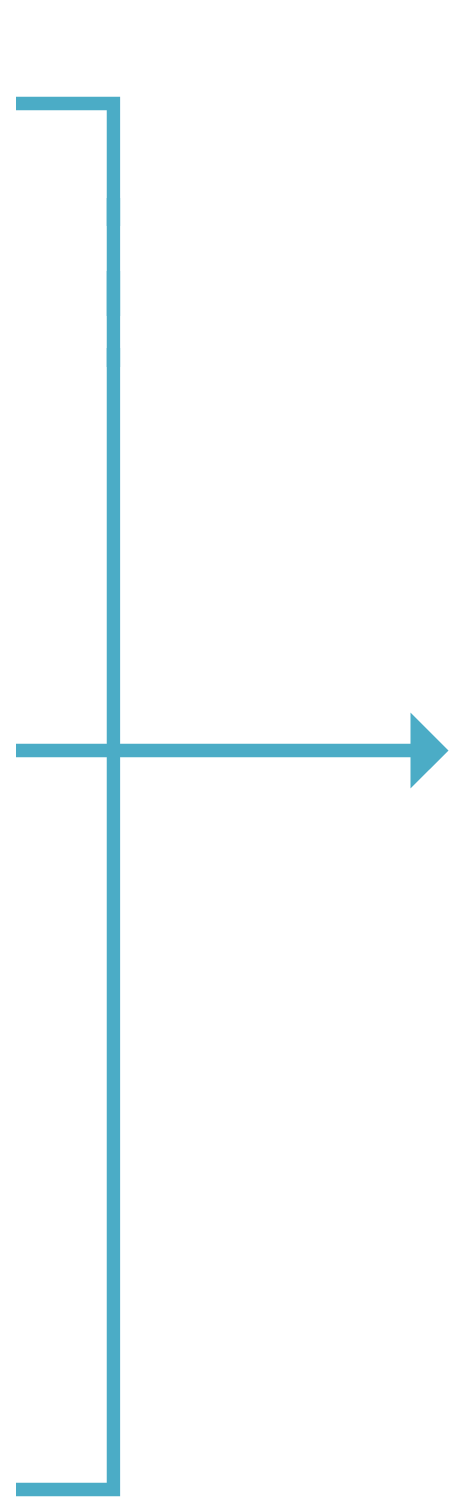
Uses real world evidence to assess most effective treatment pathways based on comorbidities
Synthesises combined outputs and prioritises treatment pathways, supported by statistical analysis
learning
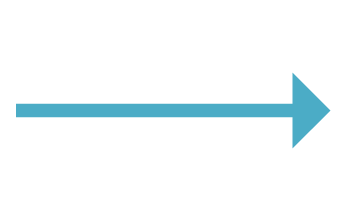
cohorts
Stratifies patients by overall clinical outcomes
Provides a nuanced approach for patients with comorbidities
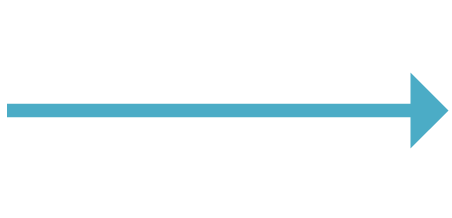
Neural networks able to provide optimal treatment recommendations based on individual profile
Neural networks clinical modules
Diabetes
Cardiovascular disease
Chronic kidney disease

Uses real world evidence to assess most effective treatment pathways based on comorbidities
Advanced learning
Stratifies patients by overall clinical outcomes
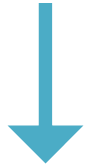
Synthesises combined outputs and prioritises treatment pathways, supported by statistical analysis
Digital twin cohorts
Provides a nuanced approach for patients with comorbidities
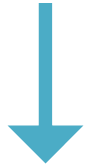
Neural networks able to provide optimal treatment recommendations based on individual profile